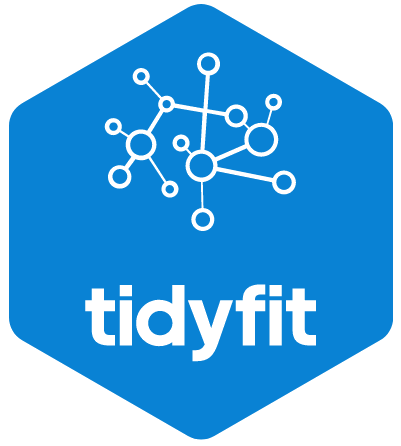
Minimum redundancy, maximum relevance feature selection for tidyfit
Source: R/model.mrmr.R
dot-model.mrmr.Rd
Selects features for continuous or (ordered) factor data using MRMR on a 'tidyFit' R6
class. The function can be used with regress
and classify
.
Arguments
- self
a 'tidyFit' R6 class.
- data
a data frame, data frame extension (e.g. a tibble), or a lazy data frame (e.g. from dbplyr or dtplyr).
Details
Hyperparameters:
None. Cross validation not applicable.
Important method arguments (passed to m
)
feature_count (number of features to select)
solution_count (ensemble size)
The MRMR algorithm is estimated using the mRMRe::mRMR.ensemble
function. See ?mRMR.ensemble
for more details.
Implementation
Use with regress
for regression problems and with classify
for classification problems. The selected features can be obtained using coef
.
The MRMR objects have no predict
and related methods.
References
De Jay N, Papillon-Cavanagh S, Olsen C, Bontempi G and Haibe-Kains B (2012). mRMRe: an R package for parallelized mRMR ensemble feature selection.
See also
m
methods
Examples
# Load data
data <- tidyfit::Factor_Industry_Returns
# Stand-alone function
fit <- m("mrmr", Return ~ ., data, feature_count = 3)
coef(fit)
#> # A tibble: 3 × 3
#> term estimate model_info
#> <chr> <dbl> <list>
#> 1 IndustryTelcm 1 <tibble [1 × 0]>
#> 2 Mkt-RF 1 <tibble [1 × 0]>
#> 3 SMB 1 <tibble [1 × 0]>
# Within 'regress' function
fit <- regress(data, Return ~ ., m("mrmr", feature_count = 3),
.mask = c("Date", "Industry"))
coef(fit)
#> # A tibble: 3 × 4
#> # Groups: model [1]
#> model term estimate model_info
#> <chr> <chr> <dbl> <list>
#> 1 mrmr Mkt-RF 1 <tibble [1 × 0]>
#> 2 mrmr SMB 1 <tibble [1 × 0]>
#> 3 mrmr RF 1 <tibble [1 × 0]>